Automatic speech recognition (ASR) models are key to our understanding of political communication. They allow us to convert audio data into text data, so they are vital to any of our projects which involve analyzing audio communication on a large scale. However, they are impeded by poor quality audio, background music, or less common pronunciations of words, potentially resulting in unreliable results. Latine candidates are more likely than white, non-Latine candidates to have an accent or have learned English as a second language. To gain confidence in the use of transcriptions for downstream tasks, we want to evaluate the performance of current models on these candidates.
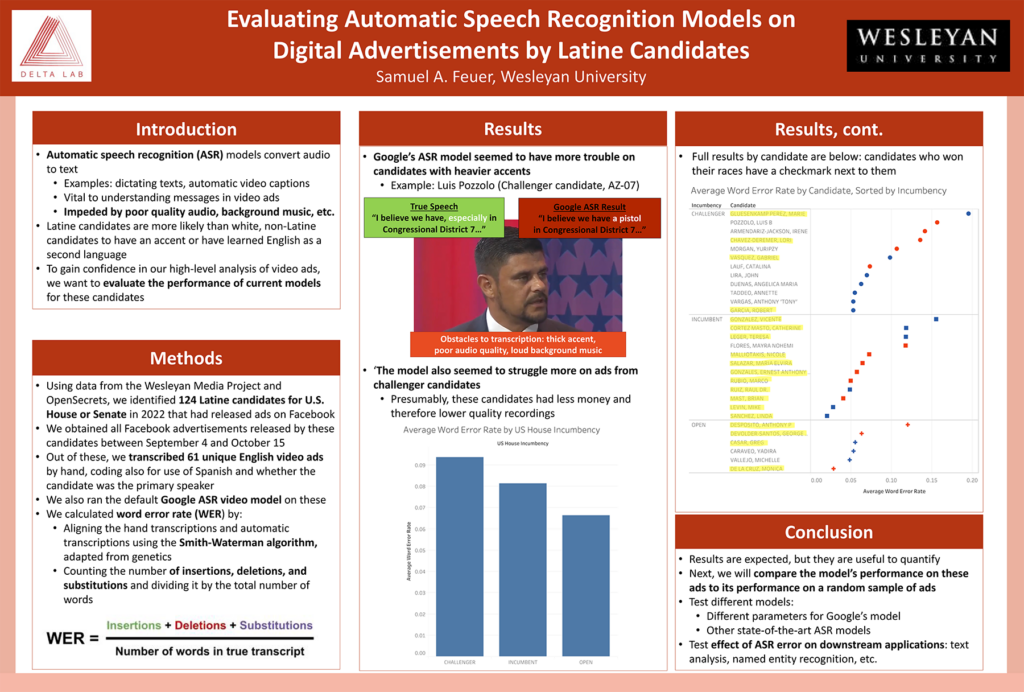
We transcribed 61 video advertisements from these candidates by hand and compared them to the Google ASR video model’s transcriptions. Our metric of comparison was word error rate, which measures the difference between two documents at the word level. We found that the Google ASR model did seem to perform less reliably on ads from candidates with heavier accents. It also performed worse on ads from challenger candidates, who may have had less money or may have been “lower-quality” candidates. Now, we are working on an extension of this project which aims to measure the effectiveness of these models on a wider range of political advertising data. Finally, we hope to measure the effect of these ASR mistranscriptions on a variety of downstream applications, including text analysis and named entity recognition.
Check out more student posters:
• Facebook Ads and Campaign Styles, and Issue Based Networks
• Facebook Ads and Campaign Styles, and Issue Based Networks
This material is based upon work supported in part by the National Science Foundation under Grant Number 2235006. Any opinions, findings, and conclusions or recommendations expressed in this material are those of the author(s) and do not necessarily reflect the views of the National Science Foundation.