By Ori Cantwell ‘22, Dale Ross ‘22, Emma Tuhabonye ‘23
Abortion has emerged as a key polarizing issue for voters over the last few decades. Attitudes toward abortion predict voters’ decisions across levels of government––presidential, congressional, gubernatorial, lower offices––making abortion a matter of issue ownership for political parties (Jelen & Wilcox, 2003). Since the pro-life movement gained political traction in the 1980s, media attention on pro-choice vs. anti-abortion interest groups has consistently (a) linked the groups to distinct parties and (b) amplified party-specific positions in the mind of the American electorate (Carmines & Wagner, 2010). As such, pro-choice has become synonymous with the Democratic Party and anti-abortion with the Republican Party. In addition, long-term exposure to Facebook political advertisements about abortion and women’s healthcare may impact voter turnout in competitive congressional districts, particularly among women voters (Haenschen, 2022). The national conversation on abortion has become increasingly heated in the past election cycle, and abortion will only become a bigger issue when the Supreme Court rules on modifications to 1973’s landmark Roe v. Wade case during the upcoming 2022 midterm election cycle (Hulse, 2021).
We were interested in exploring the conversation around abortion rights happening through political ads, starting with language differences between pro-choice/pro-Democratic vs. anti-abortion/pro-Republican entities’ ads. Thus, we used network analysis to explore the relationships between abortion-related ad pages and sentiment analysis to examine the content of the ads themselves. We ultimately found differences between the groups’ language used in abortion-related ads.
DATA AND METHODS
Our data consisted of political advertisements related to abortion that were run during the end of the 2020 general election cycle (09-01-2020 to 11-03-2020) on Meta platforms (Facebook, Instagram, etc.).
We filtered for ads in our time period of interest containing keywords relating to abortion (abort*, fetus, fetal, pro life, pro choice, reproductive, right to life, save babies, pregnan*, anti life, anti choice, baby, babies, defend life, roe v wade, and roe).1 We then removed non-word text strings, URLs, and number strings from the data before merging in sponsor classifications from the Wesleyan Media Project/Open Secrets. Variables of interest include party_all (party affiliation of the sponsor), sponsor_name (cleaned variable for the entity), pd_id (unique entity identifier), ad_id (unique ad identifier), ad_creative_body (advertisement body text). Our final dataset was composed of 12,084 different ads related to abortion. No limiting or filtering was done to get down to this number. Of those, 3,800 had verified and complete party data. We dropped all entries from parties that were not Democrats or Republicans (party_all == other) to use for our topic modeling and sentiment analysis.
We used three approaches to understanding the language of abortion-related political ads: network visualizations, a Latent Dirichlet Allocation model, and an informed Dirichlet model. All approaches began with the full, cleaned dataset.
Network visualizations
The network visualizations to explore both words used and entity similarity within the dataset. First, we selected observations from the 50 entities with the largest quantity of unique ads, excluding neutral or non-political entities (e.g., a wildlife rescue group gathering donations for baby bears). We did this to ensure the clarity of our network graphs, as larger datasets can create a “hairball” of overlapping edges and nodes which obscures most of the graph. Within the top-50 entity group, the median number of abortion-related ads for each entity was 19.00 (IQR=12.25, 36.50) and the mean was 28.08. Entities were hand-coded as pro-choice or anti-abortion based on cues from their names (e.g., “Kansans for Life”) or information from their websites. We refer the pro-choice and anti-abortion categories as position groups. The anti-abortion position group was made up of 713 ads (49.90% of all ads) from 22 entities , and the pro-choice position group was made up of 716 ads (50.10% of all ads) from 28 entities. To compare similarity in the words used by position groups and entities, we tokenized the ad text by ad sponsor using tidytext, then used the widyr pairwise co-occurrence function to find co-occurrences between words among entities. This process of finding the tokens with the strongest mutual relationships allows us to see which position groups have consistent messaging between entities. Then, we used ggraph to visualize the pairwise co-occurrences for anti-abortion and pro-choice entities, filtering for words co-occuring in ads from more than 10 entities. Lastly, we used the widyr pairwise correlation function to find correlations between unique word-entity combinations and used ggraph to visualize the pairwise correlations above 0.20 by entity, with node sizes indicating centrality in the network. All network visualizations use a Kamada-Kawai layout, meaning the nodes are positioned to minimize the Euclidean distance between them.2
Topic modeling
We created a Latent Dirichlet allocation model, where each topic has a mixture of words. This allowed documents to overlap instead of being separated into discrete groups. Using R, we used the LDA function from the topicmodels package and set k=2 to create a two topic LDA model. We chose 2 topics because we wanted to hopefully separate into pro-Republican entities and pro-Democrat entities. We fit a topic model to estimate the topic word and document topic probabilities. Beta represents the probability of the term being generated from that topic.
After getting our results from the Latent Dirichlet allocation model we moved into the text analysis. First, we converted our data into a document-term matrix. The matrix had one row for each document and one column for each unique term. A contingency matrix where each column represents a unique vocabulary term in the corpus was created. Each row represents the count of that term in the given category. With the contingency table we can calculate the term-category associations with the pointwise mutual information (pmi) function. The pmi calculates a number of information theoretic quantities on a given contingency table. The formula for pointwise information is . A positive PMI suggests that there is word co-occurrence while a negative PMI suggests words are co-occurring less than we expect by chance.The pmi function calculates the pointwise mutual information of terms and categories, the most and least distinctive terms, and the most and least salient terms. From the pmi function “salient” and “distinctive” terms are determined. Distinctive terms appear almost uniformly across all categories. Salient terms are distinctive and also appear frequently in the corpus relative to the other terms.3
RESULTS
Network Visualizations
Word co-occurance network graphs revealed differences in language between ads from anti-abortion and pro-choice entities. As shown in Figure 1.1, anti-abortion entities’ ads had a small set of shared words, with 170 word pairs occurring in ads from more than 10 entities. The word pairs with the highest co-occurances among entities were life and abortion (21 out of 22 entities), pro and life (19), pro and abortion (19), abortion and babies (19), life and babies (18) and pro and babies (16). From this, we can see that the shared language of anti-abortion entities includes references to a pro-life stance, babies, and abortion itself. In contrast, pro-choice ads had a larger set of shared words, with 472 word pairs occurring in ads from more than 10 entities (see Figure 1.2). The word pairs with the highest co-occurrences among entities were care and health (23 out of 28 entities), health and reproductive (23), fight and reproductive (23), fight and protect (22), care and reproductive (22) and access and reproductive (22). Here, we can see that the shared language of pro-choice entities includes reproductive health care and potential reactions to anti-abortion sentiment.
A network graph of word correlation between entities showed clustering of anti-abortion and pro-choice position groups (see Figure 2). Almost all pro-choice entities appeared as a cluster on the left side of the graph, with the larger node size of NARAL, Planned Parenthood, and ACLU entities indicating higher centrality in the network. Anti-abortion entities also clustered on the right side of the graph, and the larger node size of several entities (including Women Speak Out PAC and American Life League) indicated higher centrality in the network.
Figure 1.1: Words co-occuring in ads from more than 10 anti-abortion entities
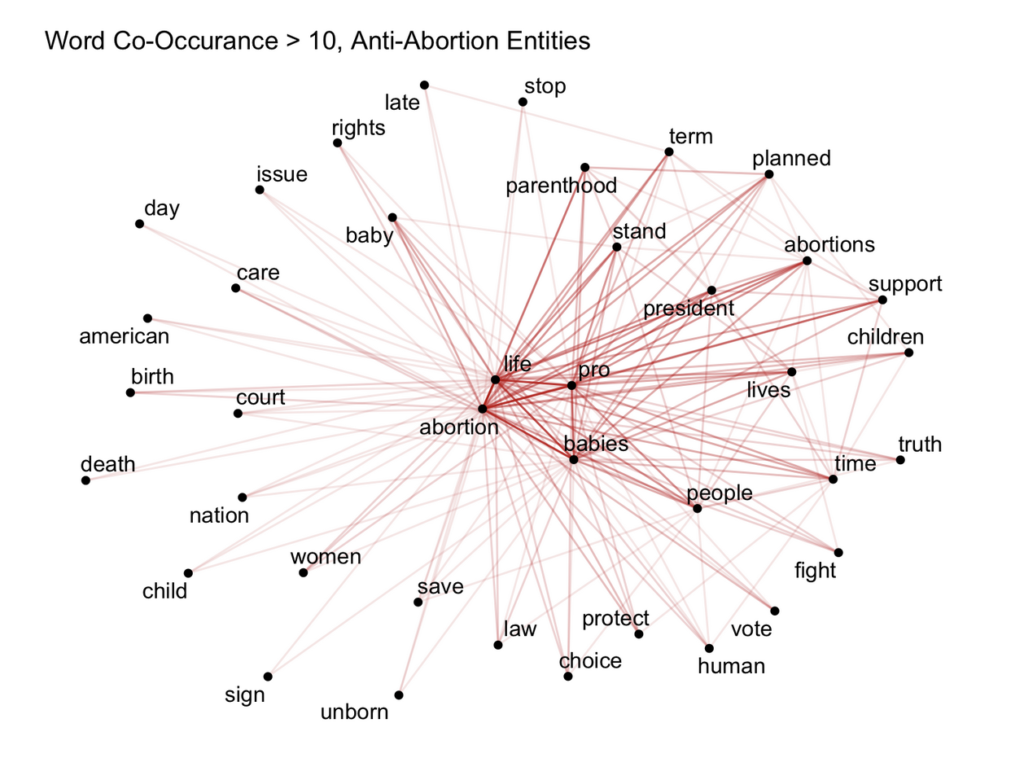
Figure 1.2: Words co-occuring in ads from more than 10 pro-choice entities
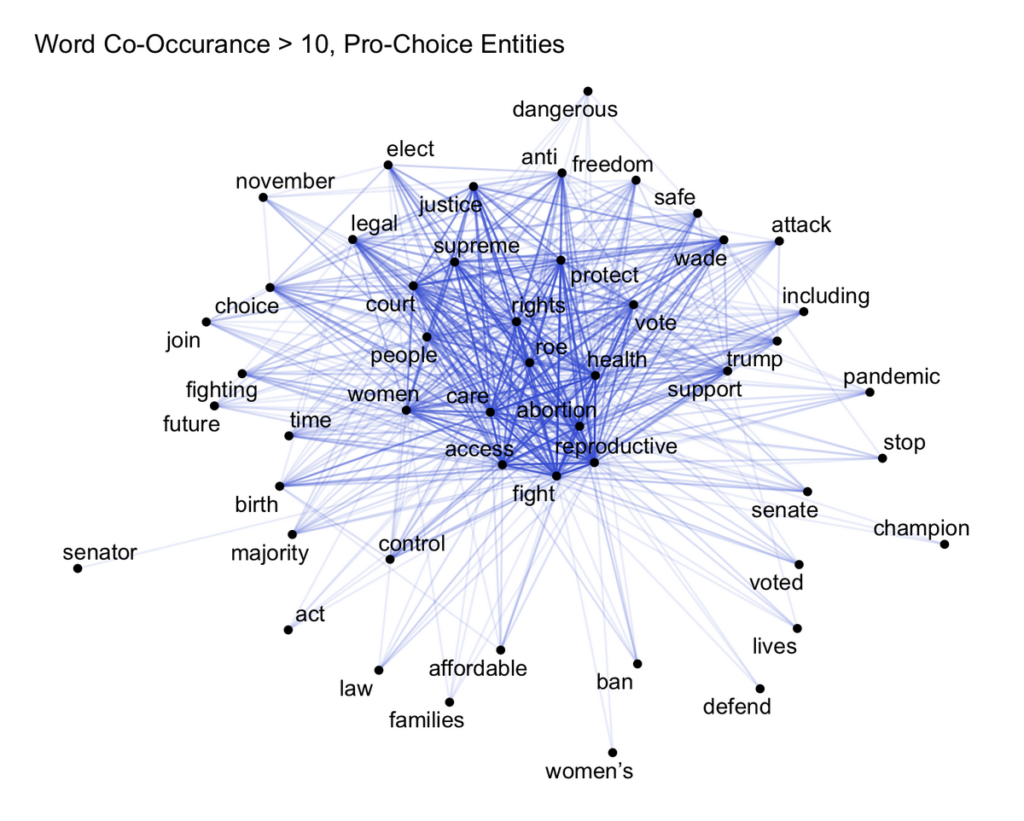
Figure 2: Word correlation > 0.2 between entities in all ads
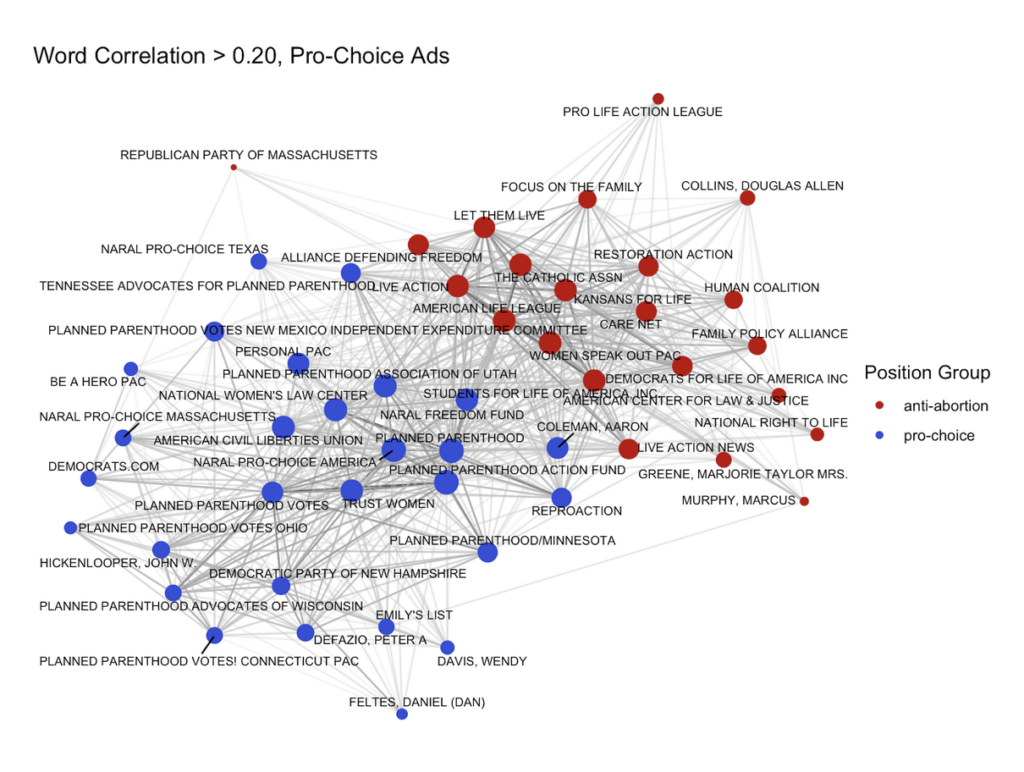
Topic modeling
The LDA topic model split the data into two topics (see Figure 4). Topic one’s top words were court, supreme, justice, ginsburg, senate, trump, and barrett. It is likely that these words mostly come from phrases like “Supreme Court Justice Ginsburg” and “Supreme Court Justice Barrett.” In topic 2, the most frequent words include right, care, need, vote, and protect. As seen in Figure 4, the LDA topic model does not show a definitive partisan divide between the two topics, but instead two rhetorical approaches within ads.
Figure 3: Sentiment analysis of all the observations
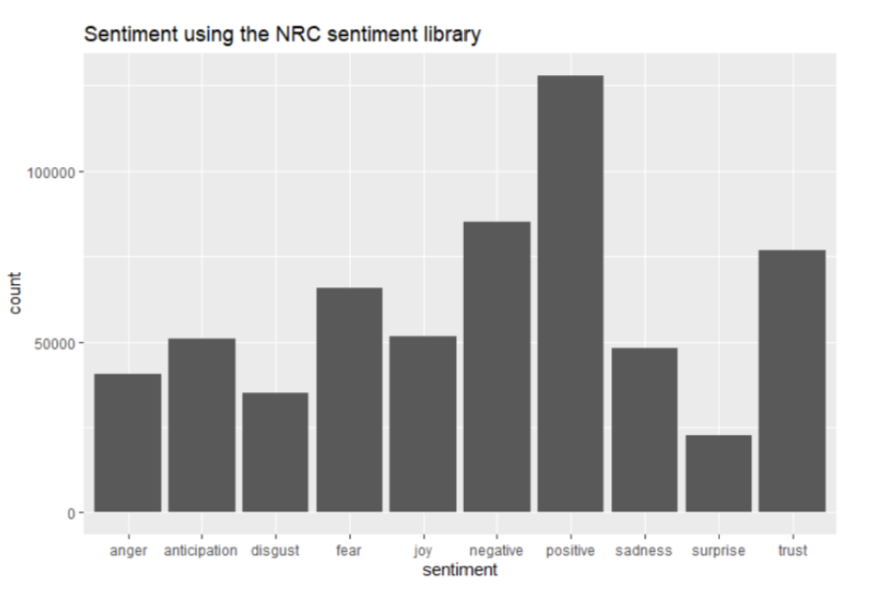
Figure 4: Latent Dirichlet Allocation model results split ads into two categories
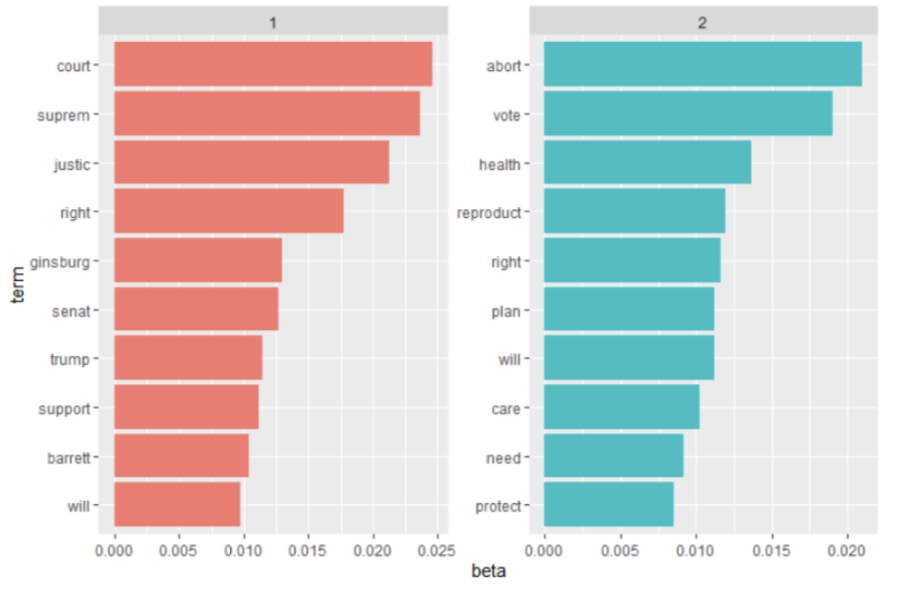
To explore the unique keywords used by pro-Democrat entities and pro-Republican entities, we then used a Chi-Squared method on the tokenized data of abortion advertisements with solely Democrat and Republican coded entries. See Figure 5 for the most unique keywords for pro-Republican entities and pro-Democrat entities, where the x-axis value is positive if the observed value in the target exceeds its expected value. Keyness results show differing keyword associations between pro-Democrat entities and pro-Republican entities.
Figure 5: Keyness results
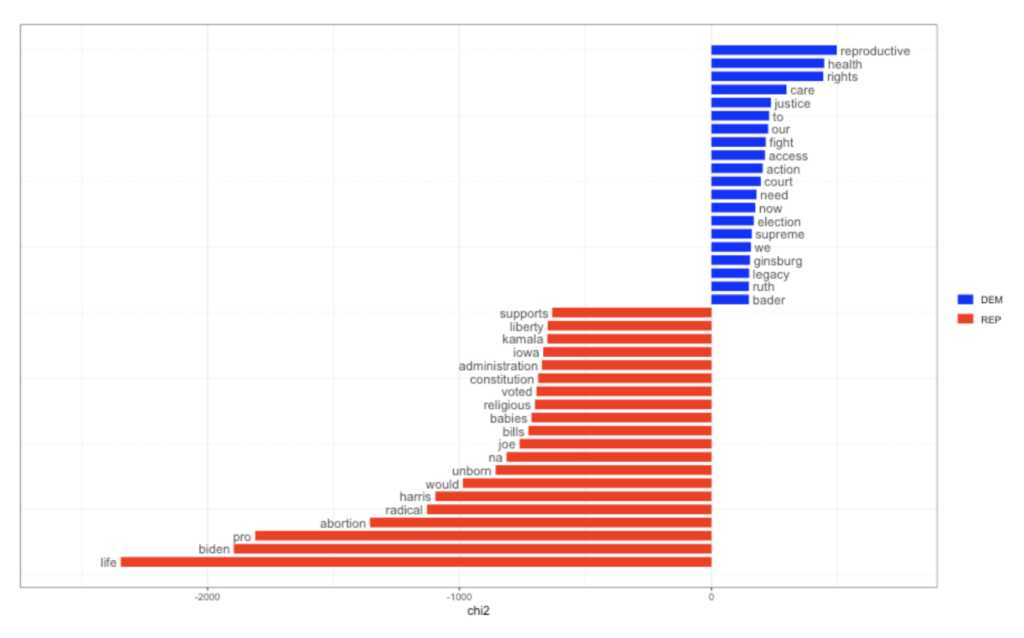
We then took these results a step further with a Fightin’ Words-style graph, which identifies meaningful words distinguishing Democrats and Republicans. The model selected is most similar to the funnel plots located in the Monroe et al. (2009) “Fightin’ Words” paper from the output of the function feature_selection(). This model includes the informed Dirichlet model. The topic words from pro-Democrat entities included ginsburg, women’s, fairness, dying, and crisis. The words that were considered most meaningful were farmers, survive, consent, unborn/born, and history.
Figure 6: Fightin’ Words style graph results
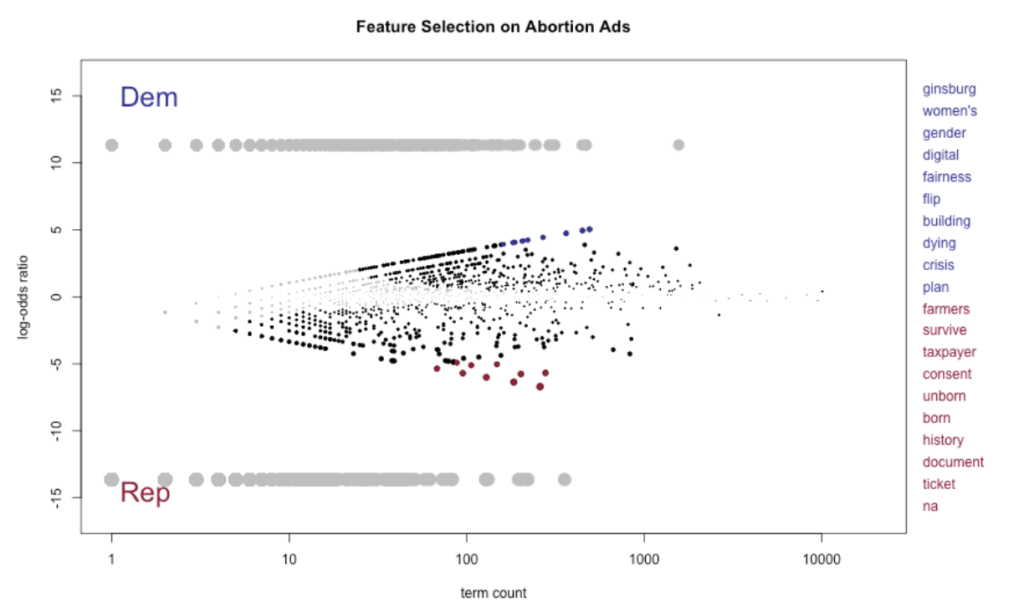
Table 1: The most common words for each party as determined by pointwise mutual information visualization scores
Top Words for Republicans | Top Words for Democrats |
---|---|
innocent | reproductive |
radical | despite |
religious | confirmation |
loeffler | couldn’t |
constitution | equality |
kill | confirmed |
liberty | lgbtq |
liberal | pressure |
joe | barrett’s |
biden’s | christy |
pelosi’s | counts |
stance | 🏳️🌈 (rainbow flag emoji) |
grabs | lgtbtq |
vp | progressive |
view | depends |
We examined the most important words for each political party using pointwise mutual information (PMI) visualization scores. Since PMI results are sensitive to infrequently occurring words, we set a term frequency threshold of at least 50 occurrences. PMI scores are also a way to analyze term association. Table 1 was gathered by the pointwise mutual information (PMI) scores and grades of the distinctness of words by pro-Democrat entities and pro-Republican entities. The SpeedReader package was used to tokenize the data where the next step was to do term-category association analysis using PMI. Our results reveal that the top three highly distinct words for pro-Democrat entities were reproductive, despite, and confirmation; the top three highly distinct words for pro-Republican entities were innocent, radical, and religious. See Table 1 for the most distinct words used in abortion ads for each party.
Conclusion
The three methods we used begin to reveal various aspects of the conversation around abortion. In comparing anti-abortion and pro-choice ads with network graphs, we found differences in the shared language used by each position group, but similarities in language used by different entities within the same position group. Within the top 50 entities we identified, we also found anti-abortion ads to have a smaller set of shared words compared to pro-choice ads. In addition, unlike anti-abortion entities, the most popular shared language of pro-choice entities does not include the word “abortion.”
Topic modeling showed two types of rhetoric used in abortion-related ads: legal language (court, supreme, justice) and emotional language (care, need, protect). Sentiment analysis indicated that the top three sentiment categories were positive, negative, and trust while the least recurring sentiment category was suprise. PMI results show that pro-Republican entities’ ads’ highly distinct words include negative words used to attack pro-Democrat entities, whose highly distinct words were less divisive and more identity-based (e.g. lgbtq). The keyness results similarly suggest that anti-abortion position groups are using Democratic figures to attack pro-choice position groups, with highly unique words used by Republican groups (e.g., biden, harris, radical, joe, kamala). Once again, pro-Democrat entities tend to use less divisive language among unique words, instead focusing on more action and policy-driven words (e.g., rights, justice, fight, access).
Indeed, our findings indicate differences in language used between Democrats and Republicans when it comes to discussing abortion. Still, we are presented with several limitations in applying our results. We would like to further investigate the results of the Fightin’ Words style graph––as of right now, the data does not match our other results from our sentiment analysis and aggregation of the top words. Pro-choice entities also funded more ads during our time period of interest, so a majority of ads in this analysis are pro-choice. In future research, we would like to include more data from anti-abortion entities to get a balanced view of the language used to discuss abortion.
Notes
1 The keywords were applied inclusively, meaning “pregnancy” and “pregnant” would both be captured by pregnan*, but not slang like “preg” or “preggo.” We allowed up to five characters between words, meaning “pro-life” and “save the babies” would be captured by pro life and save babies, respectively, but irrelevant phrases like “professional life” or “save money for your grandbabies” would not be captured.
2 For further details on the Kamada-Kawai algorithm for network graphs, see: Kamada & Kawai (1989)
3 www.mjdenny.com/getting_started_with_SpeedReader.html
Bibliography
Carmines, Edward G., Jessica C. Gerrity, and Michael W. Wagner. “How Abortion Became a Partisan Issue: Media Coverage of the Interest Group‐Political Party Connection.” Politics & Policy 38.6 (2010): 1135-1158.
Haenschen, K. (2022). The Conditional Effects of Microtargeted Facebook Advertisements on Voter Turnout. Political Behavior, 1-21.
Hulse, Carl. “Abortion Decision Could Spill into Midterm Elections.” The New York Times. The New York Times, December 2, 2021. https://www.nytimes.com/2021/12/01/us/abortion-midterm-elections-supreme-court.html.
Jelen, T. G., & Wilcox, C. (2003). Causes and consequences of public attitudes toward abortion: A review and research agenda. Political Research Quarterly, 56(4), 489-500.
Kamada, T., & Kawai, S. (1989). An algorithm for drawing general undirected graphs. Information Processing Letters, 31(1), 7-15.
Monroe, B. L., Colaresi, M. P., & Quinn, K. M. (2008). Fightin’ Words: Lexical feature selection and evaluation for identifying the content of political conflict. Political Analysis, 16(4), 372-403.